ANR JCJC GraVa (2019-2023) id: ANR-18-CE40-0005
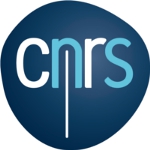
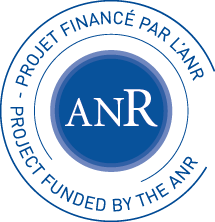
Presentation
Handling large datasets has become a major challenge in fields such as applied mathematics, machine learning and statistics. However, many methods proposed in the literature do not take into account the fine structures (geometric or not) behind the underlying data. Such structures can often be modeled by graphs.
Though many worldwide companies such as Google, Facebook or Twitter, have made their success extracting information where the signals live natively on a graph, a refined analysis of the underlying graph influence is still missing and most of the literature neglects, for simplicity, the underlying graph structure, or uses over simplistic linear estimators to overcome these issues. We advocate the use of robust non-linear regularizations to deal with inverse problems or classification tasks on such signals.
Project GraVa is an endeavor to solve such concrete and difficult issues through the mathematical perspective of variational methods for graph signal processing. This stance raises several challenges:
-
Which estimators are good candidates for such tasks, and how to assess their performance? We will encompass recent contributions from communities of graph harmonic analysis, statistics and optimization, and develop new tools in nonlinear spectral graph theory which is only emerging.
-
How to design computationally tractable algorithms for these methods? We will rely on modern distributed and parallel optimization schemes. Our main ambition is to go beyond standard approaches by fully taking advantage of key graph properties (regularity, sparsity, etc.).
-
What is a good metric to compare graph signals and how to classify them? Measuring an L2-error is standard in signal processing but reflects a Gaussian assumption on the noise distribution. We intend to develop more robust and structure-dependent error metrics able to deal with inverse problems, segmentation and classification tasks.
-
How to tackle time-dependent signals? Dealing with static signals is a first step, but many networks requires considering time-evolving graphs structures. We plan to transfer time-series analysis to graph signals in order to deploy our achievements to real case scenarios.
Members
Permanent members
- Charles Deledalle, previously CNRS & Université de Bordeaux, now BrainCorp
- Joseph Salmon, Université de Montpellier
- Xavier Dupuis, Université de Bourgogne
- Nicolas Tremblay, CNRS & Université de Grenoble-Alpes
- Samuel Vaiter (PI), CNRS & Université de Bourgogne
PhD student
- Quentin Klopfenstein, Université de Bourgogne
- Hashem Ghanem, Université de Bourgogne
Organized events
- Optimization for Machine Learning, March 9-13, 2020, Marseille, France.
- Graph signals : learning and optimization perspectives, May 2-3, 2019, Montpellier, France